The Road to Identity-First Security: Identity Data & Observability
- John Pritchard
- March 7, 2023
- Identity Data Fabric
- 6 MIN READ
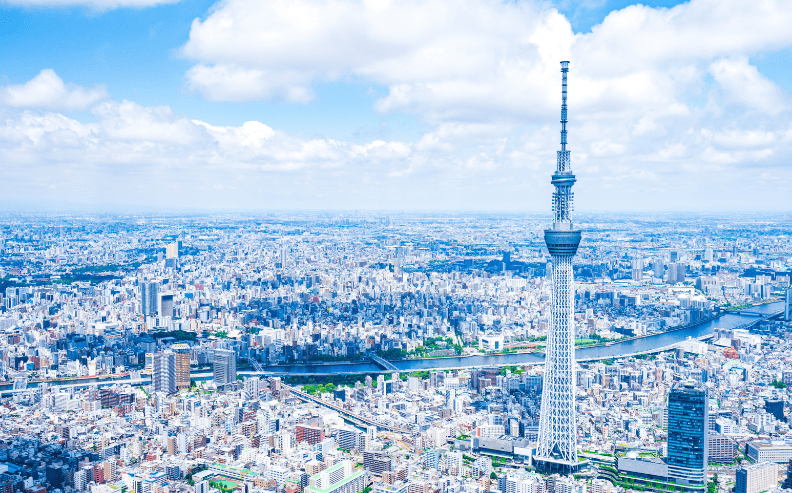
Decentralization of Identity Data
As organizations move workloads out of the network and into the cloud, they are replacing traditional perimeter defense security techniques with a Zero Trust approach, bringing Identity to the forefront. Today, Identity and Access Management (IAM) teams are adopting numerous access control measures such as step-up authentication, privileged access management, least privilege provisioning, and fine-grained authorization to secure access to the network, resources, and applications. This growing number of controls adds defensive layers but also decentralizes decision-making across multiple policy enforcement points.
IAM strategies are changing from being completely centralized to having a combination of centralized policy management and governance, and decentralized policy enforcement. For example, an administrative policy can be executed by an Identity Governance and Administration (IGA) tool that manages entitlements, while a runtime policy can be executed by an access management tool that authorizes access to a resource.
Common to all policy engines is the need for identity data to evaluate every authentication and authorization decision. Getting the right identity data to the right places at the right time enables better risk-based access decisions. However, identity data itself is typically highly decentralized, existing in directories, databases, and SaaS applications across the enterprise. Differing user constituency requirements (employees, contractors, customers) and identity store deployments (on-prem and cloud) mean no single identity data repository exists.
While there have been notable advances in the areas of policy orchestration (employee, customer and IoT on-boarding journeys) and policy management (Open Policy Agent and fine-grained authorization), there has been much less focus on the role of policy information–the underlying identity data. When identity data issues occur—such as partial, inaccurate, or stale data, or even an unauthorized change—the impact can rapidly multiply and escalate in an environment with multiple policy decision points. These data incidents can have significant consequences and multiple negative repercussions from lack of trust to loss of revenue. A lack of visibility into data quality can also produce false positive indications or faulty insights, which can lead the organization to make poor decisions.
At the end of the day, every application and decision point wants to go to one place to get all the identity data it needs to perform its function in exactly the format, structure, schema, and protocol that it needs. A simple starting point is to integrate distributed identity data via a series of authoritative identity data pipelines, weaving together identity attributes into an Identity Data Fabric that is bi-directionally synced on a regular basis. Its purpose is to deliver accurate, flexible, reusable, and consistent identity data to policy engines across the entire IT landscape.
The Need for Identity Observability
Identity data pipelines alone do not equal good policy enforcement. High quality, governed policy information is essential for policy engine execution. You need the right data for the right decisions.
Identity Observability is the ability to assess the health and usage of all identity data within an organization regardless of where it is stored, and gain visibility into health indicators of the overall identity infrastructure.
By using a combination of attribute and schema changes with time-series analysis, an observer can interpret the health of datasets and pipelines, identifying risk areas that undermine policy engine execution. Identity Observability enables identity teams to set monitors and be alerted about data quality issues across the overall system. What is key here is the emphasis on quality in addition to uptime.
Why is this important? Because while your infrastructure may look fine and your identity data pipelines are operational, your underlying identity data may have changed in a way that changes the outcomes of your distributed policy engines. Thus, to ensure that the identity governance you are performing is accurate and valuable, you need to observe the data, the pipelines, and the infrastructure at the same time.
Within your Identity Data Fabric, you need to gather metadata attributes that allow you to assess data quality, namely timeliness, completeness, distribution, schema, and lineage. This can be described as data observability. Once aggregated, this data can be analyzed with advanced AI and ML processes to identify patterns, outliers, associations, remediations, and cross entity relationships previously hidden in the data.
Additionally, identity data pipelines that federate backend data sources need to be monitored for changes in volume, behavior, drift, and uptime. This can be described as pipeline observability.
Lastly, you need to monitor the underlying execution environment for compute, storage, and networking health and performance. This is infrastructure observability.
The main goals of Identity Observability are to minimize “time to insight,” which is the time to understand what is happening in the system and “time to resolve,” the time to resolve quality of service issues.
Identity Observability Metadata
Identity Observability relies on automated sourcing, identification, analysis, insight, logging and tracing of identity data, data pipelines and identity infrastructure to evaluate data quality and identify issues. To generate this type of end-to-end data observability, it is necessary to collect, record, and trace specific identity metadata throughout your environment:
- Timeliness: Recording when identity data is created, updated, or deleted allows you to determine how current the data is and if it needs to be refreshed. In a highly distributed environment, it is common for data synchronization to occur in batch or within synchronization windows. Observing timeliness allows you to evaluate identity data as time series changes. Ideally, when possible, identity data changes can be captured, analyzed, and applied in near real-time to ensure the most accurate policy decisions.
- Completeness: Missing values indicate that no data value is stored for the attribute in an observation. Understanding incomplete data is important because missing values can add ambiguity and result in inaccurate decision making or complete process breakdown. Observability provides the opportunity to not only identify critical gaps in data values, but potentially automate remediations.
- Distribution:
- Analyzing the distribution of identity data is a powerful data science technique to uncover unexpected changes and anomalies. Distribution refers to the shape of the data. It can be the frequency of a category, i.e., the number of occurrences, or the descriptive statistics of numerical values (average, mean, standard deviation).
- Distribution metrics allow organizations to establish baselines from which change can be evaluated. Extreme variance in data can indicate accuracy concerns. Distribution measures can identify anomalies that may indicate an unexpected change in your data source or monitor for changes that fall outside an expected range.
- Volume: Logging the amount of identity data that is transiting through pipelines allows you to define baselines and determine whether your identity data source generation is meeting expected thresholds or exhibiting anomalous behavior. Understanding the scale of the identity data at work in the environment provides insight into proper sizing of other components in the IAM and ZTA architecture.
- Schema: Schema analysis monitors for changes in the underlying data structure. Numeric data suddenly represented as text data, or a flattening of hierarchical relationships will impact downstream clients. Combining schema change metadata with other observations such as volume, allows teams to evaluate the impact on dependent systems. Considering how many clients access a particular view (volume metric) that has had a data attribute change (schema) allows organizations to make informed decisions about impact and remediation.
Beyond Policy Engines, Data Observability is Important to AI/ML
Artificial intelligence (AI) and Machine learning (ML) are potential game changers for securing the enterprise. The ability to infer risk based on behavior sets the stage for organizations to implement real-time and adaptive controls. AI/ML in IAM, however, is still very much in its infancy. The prevalence of false positives or opaqueness of underlying risk engines have yielded skepticism. AI/ML depends upon data to feed its models. The reliability of that data, more so than the amount, can directly impact the success of those models. The issue is not more data, but better data. Even small-scale errors in training data can lead to large-scale errors in the output.
Although incomplete, inconsistent, or missing data can drastically reduce AI/ML prediction strength, timeliness metadata—more so than any other type of data—is the greatest concern in developing AI/ML models. A complete and accurate, yet stale, data set will lead to a prediction model trained to interpret behavior of the past. Implementing practices like Identity Observability dramatically improves the potential accuracy of AI/ML risk signals.
Next Steps
Zero Trust, Identity-first strategies are driving decentralized policy enforcement. Identity data engineering solutions like Identity Observability ensure policy engines have the right data for the right decisions. Even organizations at the beginning of an IGA initiative are well served addressing their data issues first and setting a solid foundation to serve dynamic runtime authorization.
So, what do you think? I encourage you to reach out and contact us, let us know what you envision your organization doing with Identity Observability in your future. Or, if you’d rather see for yourself get your hands on our RadiantOne Trial, anytime.
Explore more
Subscribe to receive blog updates
Don’t miss the latest conversations and innovations from Radiant Logic, delivered straight to your in-box.